Generative AI is transforming sentiment analysis, enabling businesses to extract nuanced insights with unprecedented flexibility and accuracy. Traditional approaches to sentiment analysis often rely on rigid classifications and predefined structures. However, new AI models are expanding these boundaries, making sentiment analysis more adaptable to context, complex language, and industry-specific jargon.
In this blog, we’ll explore how to leverage generative AI for sentiment analysis, why it’s a game-changer, and how to get started.
How Generative AI Differs from Traditional Approaches
Unlike traditional sentiment analysis, which tends to be more rigid, generative AI enables a more nuanced understanding. In particular, generative AI can capture:
- Subtle Sentiments: It interprets ambiguous emotions, such as mixed feelings or sarcasm, which traditional models often miss.
- Contextual Understanding: It considers the context, not just isolated words, leading to more accurate interpretations.
- Industry-Specific Nuance: It adapts to unique terminology across different domains, such as customer support, finance, or healthcare, making sentiment analysis more relevant to specific use cases.
Steps to Conduct Sentiment Analysis with Generative AI
Generative AI opens up new methods for conducting sentiment analysis, shifting from rigid classification to dynamic interpretation. Here’s a step-by-step guide:
Step 1: Collect and Preprocess Data
Gather textual data from relevant sources, such as:
- Customer reviews, social media comments, and open-ended survey responses
- Chat logs from customer support or product feedback channels
- Industry-specific sources, such as financial news or clinical patient feedback
If the data includes a mix of different languages, AI tools can easily translate it into English. Additionally, AI can even help flag unstructured text data that may have been generated by AI, which is becoming increasingly common.
Step 2: Choose the Right AI Model
Selecting the right model is crucial. Different AI models vary tremendously in performance. Consider three factors when making your choice: performance, price, and speed.
In terms of performance, as mentioned, there can be a huge difference from model to model. For example, the latest GPT-4 model demonstrates exceptionally high performance, while models like GPT-3.5 may lag significantly. Therefore, if the quality of sentiment analysis is critical, opt for the top-performing models.
Regarding price, the best models, such as GPT o1 models, can cost significantly more. This is not only due to a higher token cost but also because they consume more reasoning tokens than other models.
Finally, speed is generally less of a concern since most models process data much faster than if you do it manually.
Step 3: Develop the Most Optimized Prompts
The power of generative AI in sentiment analysis often lies in the prompt. Craft specific prompts to elicit nuanced understanding. For example:
- Basic Sentiment Query: “Analyze this review for sentiment and summarize its tone.”
- Contextual Analysis: “Identify the main sentiment in this social media comment, noting any sarcasm or mixed feelings.”
- Industry-Specific Sentiment: “Analyze the tone of this customer support email regarding a technical issue and identify the underlying level of customer satisfaction.”
When building your AI prompts, two factors can significantly enhance the quality and accuracy of your sentiment analysis:
- Add Project Context and Goals: Providing background information helps AI interpret responses more accurately.
- Clear Questions: Ensure your question is written clearly, emphasizing that the query is about sentiment.
Example Use Cases
Here are some ways generative AI enhances sentiment analysis across industries:
- Customer Service: Generative AI can analyze chat logs to detect frustrated customers, generate empathy-driven response templates, and alert service teams to issues needing escalation.
- Social Media Monitoring: By interpreting nuanced language, generative AI can track brand sentiment over time, identify influencers, and provide in-depth summaries of public opinion on specific campaigns.
- Healthcare and Patient Feedback: AI models can detect sentiment in patient feedback, interpreting emotions like anxiety or satisfaction to help healthcare providers improve patient experiences.
- Financial Services: Analyzing sentiment in financial news or investor feedback provides insights into market sentiment, helping analysts gauge public opinion on financial products or company performance.
BTI Platform: In-Depth and Accurate Sentiment Analysis with AI
The BTI platform enables you to analyze thousands of open-ended text responses into sentiment themes in seconds, offering full flexibility to review and edit the themes.
It also highlights specific quotes for each theme, providing comprehensive, in-depth quotes that enrich your analysis.
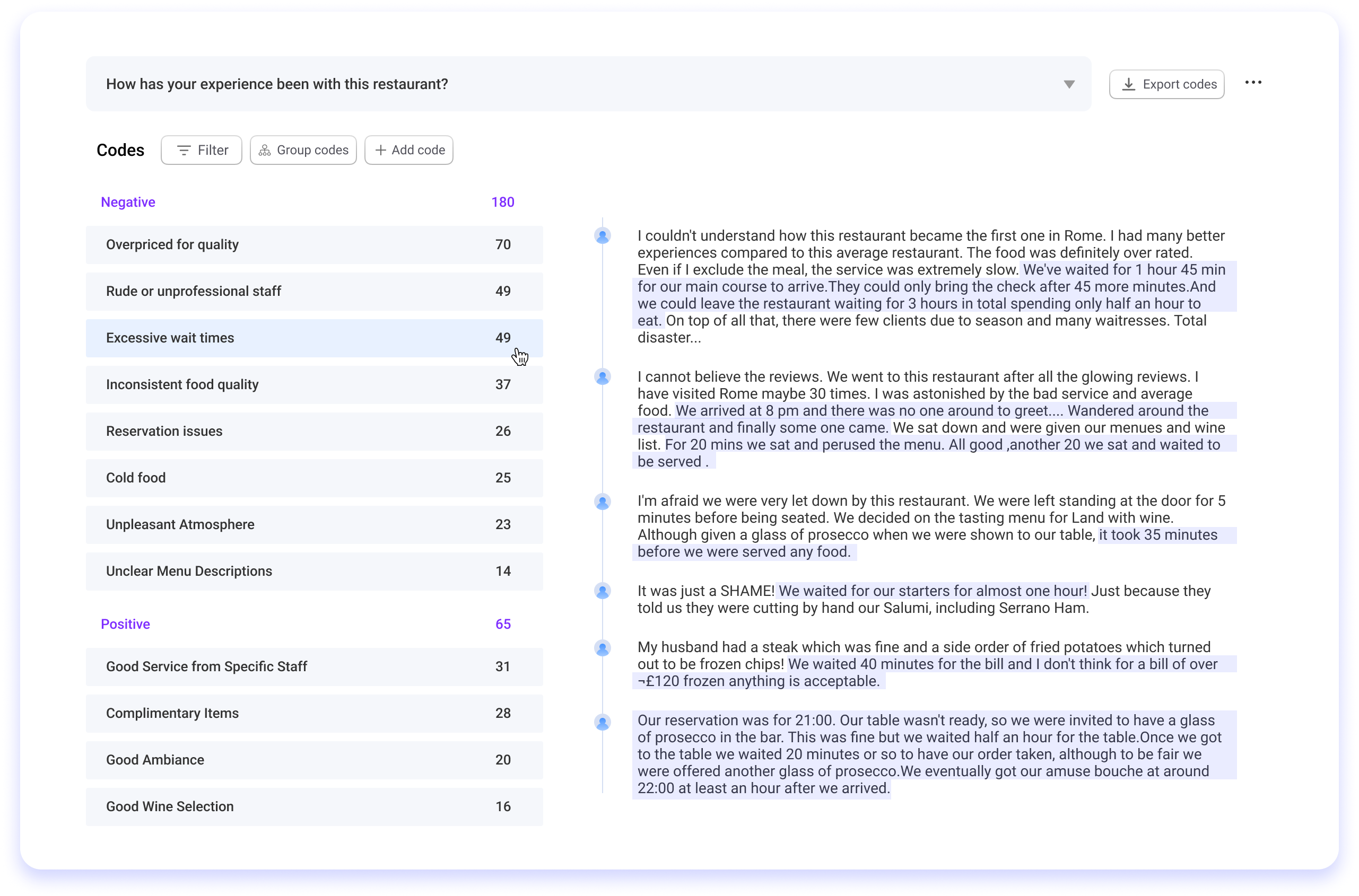